Enterprise Architect
Ever-increasing demand for traditional and novel data needs is fueling a new trend in corporations: the data marketplace. Within this new trend, there is a significant need for focus and attention on basic data architecture, data stewardship, and data governance around enterprise data assets. Organizations of all sizes are pursuing adoption of leading data transformation strategies, but many of these visions are absent of practical data governance strategies. The strategies and disciplines defining business definitions, architecting, and modeling data, especially data governance, have become even more important to the success of any data transformation initiative.
Data Governance – Using AI for Quick Adoption
Why has data governance been viewed as a “Godzilla vs. King Kong” sized project? Why has it seemed so complicated, and the perceived effort seems unworthy of the investment and energy necessary to produce meaningful results? In the past, the commonly accepted methods for data governance were flawed and often tried to eat the whale in one bite, leading to slow and ineffective programs especially with a trend away from big data and enterprise data warehousing solutions. A new approach is in order that will facilitate quick adoption and change in the perceived value of efforts around data governance. That new approach is now centered around leading Artificial Intelligence (AI) technologies which enable organizations to implement data governance strategies quickly by adopting a domain-based approach. Bringing AI into the data governance approach is a strategic step for organizations to enter the AI and Machine Learning (ML) space in a way that will breed enthusiasm for the technology. More importantly, it will bring smiles to the face of the end-user’s community when searching for corporate data sets from a centralized, user-friendly interface where others are also searching and sharing results.
Searchable Data Catalog
In today’s data-hungry, “need it now” world, users need access to data quickly and continuously. The question that needs to be answered is, “Where can I shop for my data that will ensure my users’ appetites are met without delay?” The world of structured and unstructured data siloes, data lakes and file systems on-prem and in the cloud often lack the completeness, business definitions, overall context, and comprehensive metadata with uncertainty of the source and latency of the data. These practices are where IT organizations have fallen short, leading to frustration and mistrust of IT. To enable corporate data consumers to capitalize on use of enterprise data assets, the development of a comprehensive, business-driven searchable data catalog is needed. This data catalog can be organically grown by data domain and will provide that shopping place to satisfy all users from all areas of the business.
IBM Watson Knowledge Catalog
IBM’s Watson Knowledge Catalog (WKC), a component of IBM Cloud Pak for Data, provides such a capability. This AI-driven cataloging capability provides the ability to not only build a single searchable catalog, but multiple catalogs, meeting the various needs of different parts of the organization. Data catalogs contain business definitions, data lineage, and comprehensive metadata, and they provide the ability for intelligent searching. IBM’s “accelerators” are free community-designed solutions covering many verticals. They will supercharge a cataloging project effort by pre-loading the data catalog with meaningful business terms that can be discovered or “matched” to the organization’s specific enterprise data assets.
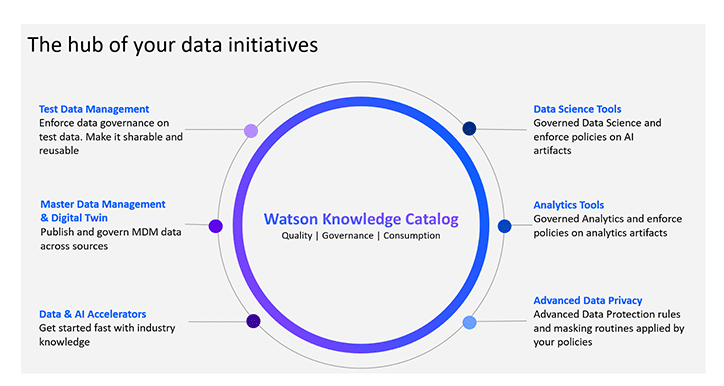
The embedded AI features of IBM’s Cloud Pak for Data include an auto discovery capability that streamlines the discovery of all the key metadata for each data asset. With the “discovery” of each data asset brought into the catalog, the AI engine becomes trained (learning) more and more about the company’s data. Over time, Cloud Pak for Data can more efficiently identify naming standards within the data to quickly match to data entities, data types, and data classes. This will also usher in the use and adoption of AI within an organization, in a way that is not overwhelming but intuitive, leveraging the latest AI and ML technologies. WKC will also enable data stewardship in a way that meets varying personas (data engineer, data scientist, business analyst, etc.) while ensuring that data governance policies around data privacy, GDPR (General Data Protection Regulation), CCPA (California Consumer Privacy Act), and other customer-defined data access rules are followed in a trackable workflow.
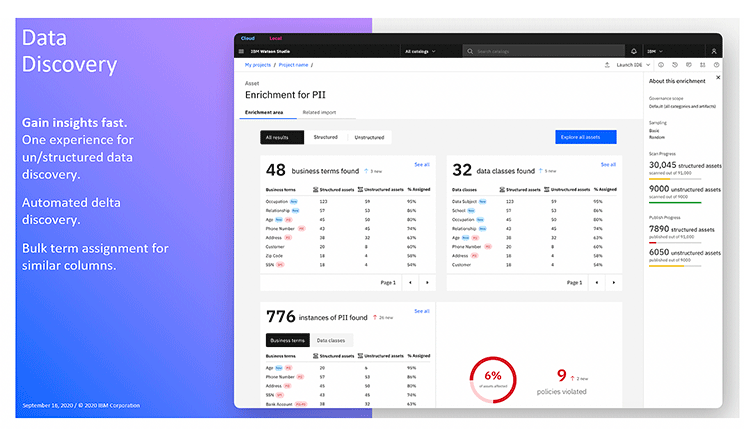
A robust data marketplace provides the end-user community the efficient and effective method to satisfy the thrust for data-intensive reporting and analytics needs. Building out a business-centric governed data catalog is a foundation component to support a successful data governance initiative.
More Information:
Mainline offers a comprehensive portfolio of data management, governance, integration, business analytics, AI, and ML solutions. For more information, contact your Mainline Account Executive directly or click here to contact us with any questions.
You May Also Be Interested In:
BLOG: What Happened to Netezza
WEBINAR ON-DEMAND: Cloud Pak for Data – Turning Data into Insights (54:32)
VIDEO: Cloud Pak for Data – Make your Data Ready for AI & Cloud (2:09)